Energy Blog: Is AI Too Power-Hungry for Our Own Good?
Energy Blog: Is AI Too Power-Hungry for Our Own Good?
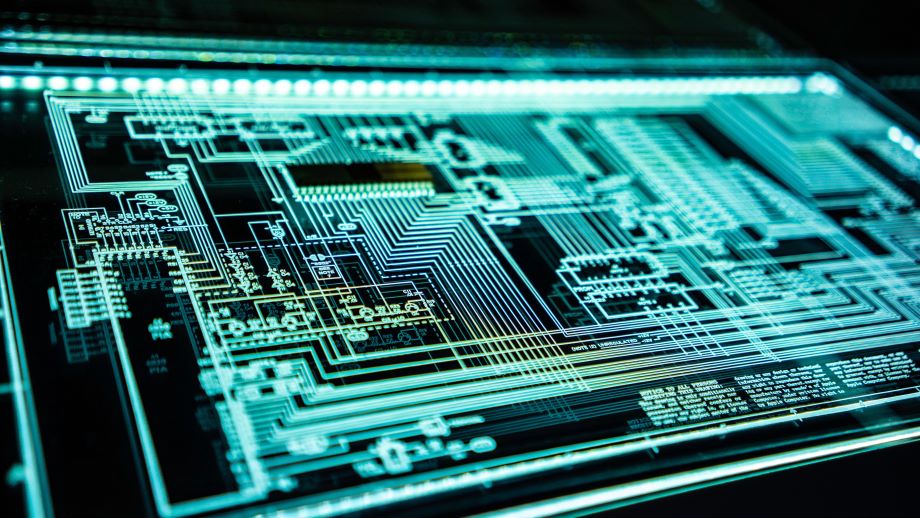
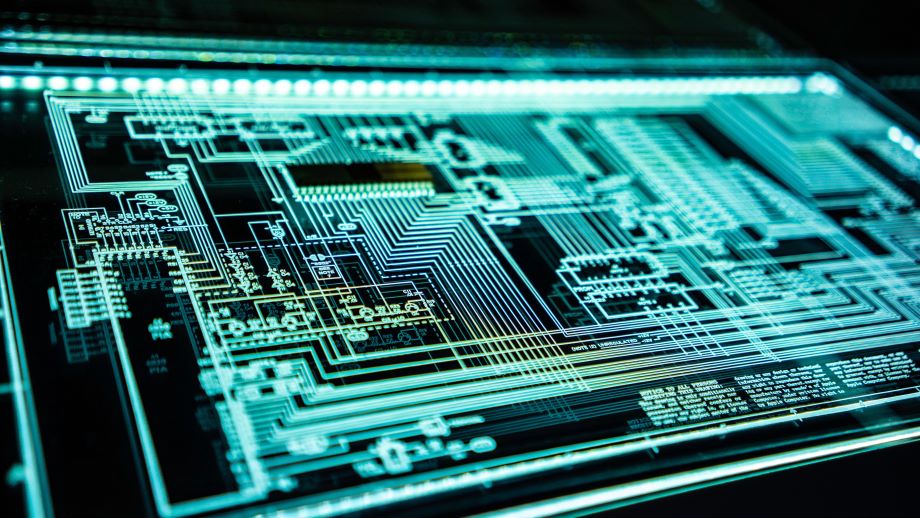
Artificial intelligence platforms may need gigawatts of electricity. But AI could offset that by unlocking new means for saving energy or producing clean power.
Editor’s note: In light of the news that the Three Mile Island nuclear plant is reopening to supply power to Microsoft for its artificial intelligence operations, we are opening access to this column by Michael E. Webber. The column first appeared in Mechanical Engineering magazine's special 2024 Summer Issue, a benefit to ASME members.
Futurists have been making bold predictions about artificial intelligence for decades, but in the last few years AI has grabbed headlines and affected the national dialog about decarbonizing the economy. The breathless claims about the rise in power consumption for the datacenters that train and host AI platforms has raised skepticism that AI’s promise will be undermined by its hunger for power.
But is the hype about power consumption real? From where I sit as a grid researcher and cleantech investor, the hype is simultaneously true and overblown. AI will surely transform society, just as the smartphone, internet, and combustion engine have done, perhaps with even swifter and more dramatic effect. In that way the hype is real. I anticipate tens of gigawatts of power plants will be required just in the USA alone just for AI (and for reference, one full-sized nuclear power plant is about 1 GW in capacity).
That sounds like a lot, but it ends up not being such a big number when compared to our other growing needs for power with the rising demand for electric vehicles and heat pumps. We are going to need a lot more electricity in the coming decades regardless of whether AI is plugged into the grid.
Having said that, one complicating factor is that AI development isn’t happening over a decades-long timescale. AI developers see themselves in a race: Speed and time-to-market is everything. That means AI developers don’t just need a lot of power, they need it now. So, the demand for power isn’t some far-away strain on the grid, it’s a here-and-now requirement that puts data centers in competition with other uses for new utility connections.
What’s more, policy makers have an incentive to support AI now and worry about the grid later. Because the power-and-chip-intensive training phase of new AI models can be done anywhere, AI companies will move their data centers to other countries rather than wait to launch domestically.
This offshoring potential from the U.S. perspective creates a national security risk; if we don’t win the race to lead on AI, then foreign adversaries will do so.
Before resigning ourselves to a world where the rush to build super-intelligent machines produces the next energy crisis, it’s worth stepping back to look at what is driving the AI energy demand in the first place.
25 to Watch: The 2024 Mechanical Engineering Watch List
AI energy demand is driven by three factors: hardware efficiency, algorithm efficiency, and scaling (that is, the number of chips devoted to AI operations). For hardware, the key efficiency metrics for AI are related to the data center itself. Power Usage Effectiveness (PUE) measures how much power needs to be delivered to the data center to provide 1 watt to the chip. Over time, PUE is asymptotically approaching the ideal: 1 watt for 1 watt.
The other measure is “FLOPS per watts,” a measure of chip efficiency. It’s a ratio of how many floating-point operations per second (FLOPS), a measure of computation speed, can be achieved per 1 watt of input power delivered to the chip. According to the Green500 list of supercomputing efficiency, the record has improved to 72.7 billion FLOPS per watt, up from 4.4 billion FLOPS per watt in 2014.
And AI algorithms may be quickly leaving their most energy-hungry stage of development. The training phase, where core models are built, is power and space intensive while being location flexible, which is why training might happen out of country. After that comes the inference phase where core models are deployed, and that does not require as much power or space.
More on Artificial Intelligence: How AI Could Impact Manufacturing
So, the good news is that hardware efficiency and algorithm efficiency are moving in the right direction. Unfortunately, AI usage has been increasing even faster, which means the growth in AI demand is overwhelming all the efficiency gains.
On the other hand, energy accounts for less than 10 percent of the costs for AI systems. The real costs are for the chips themselves and the R&D staffs to develop and train the models. And though the energy requirements for AI are non-trivial, many of the early use cases, some of which are earning revenues or achieving results, are energy related.
More from the Magazine: Crunching Numbers, Sustainably
For instance, mining and exploration companies are using AI to comb the geological literature and core samples from around the world to accelerate the discovery or copper, cobalt, and other critical minerals. Fusion startups are deploying AI to accelerate their reactor designs, research labs are using it to speed up the discovery and testing of advanced battery materials, and utilities are relying on AI to extend the deployed life of critical assets like transformers. And the petroleum industry is finding uses for AI in optimizing oil and gas networks, gas lift, and enhanced refinery asset control.
The words—accelerating, optimizing, and life-extending—are typical phrases we use to capture the potential of AI. In these ways, the energy demands by AI will be more than offset by the energy savings. Fingers crossed.
Michael E. Webber is the Sid Richardson Chair in Public Affairs and the John J. McKetta Centennial Energy Chair in Engineering at the University of Texas at Austin. Webber said he did not write this essay with AI, but he probably should have done so because in that case it would have taken less time.
Futurists have been making bold predictions about artificial intelligence for decades, but in the last few years AI has grabbed headlines and affected the national dialog about decarbonizing the economy. The breathless claims about the rise in power consumption for the datacenters that train and host AI platforms has raised skepticism that AI’s promise will be undermined by its hunger for power.
But is the hype about power consumption real? From where I sit as a grid researcher and cleantech investor, the hype is simultaneously true and overblown. AI will surely transform society, just as the smartphone, internet, and combustion engine have done, perhaps with even swifter and more dramatic effect. In that way the hype is real. I anticipate tens of gigawatts of power plants will be required just in the USA alone just for AI (and for reference, one full-sized nuclear power plant is about 1 GW in capacity).
That sounds like a lot, but it ends up not being such a big number when compared to our other growing needs for power with the rising demand for electric vehicles and heat pumps. We are going to need a lot more electricity in the coming decades regardless of whether AI is plugged into the grid.
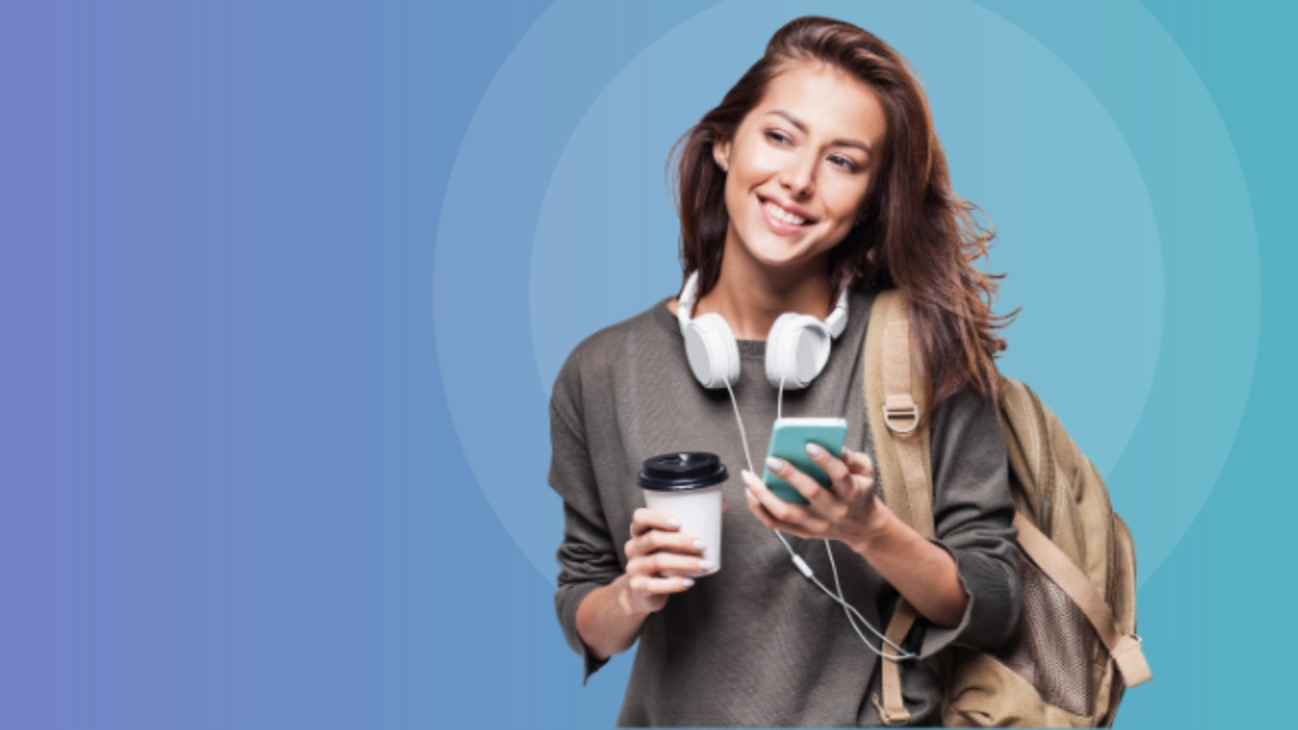
Consider the Benefits
ASME Membership provides you an instant community of engineers, opportunities to stay on top of industry trends, and a host of other valuable benefits.
What’s more, policy makers have an incentive to support AI now and worry about the grid later. Because the power-and-chip-intensive training phase of new AI models can be done anywhere, AI companies will move their data centers to other countries rather than wait to launch domestically.
This offshoring potential from the U.S. perspective creates a national security risk; if we don’t win the race to lead on AI, then foreign adversaries will do so.
Before resigning ourselves to a world where the rush to build super-intelligent machines produces the next energy crisis, it’s worth stepping back to look at what is driving the AI energy demand in the first place.
25 to Watch: The 2024 Mechanical Engineering Watch List
AI energy demand is driven by three factors: hardware efficiency, algorithm efficiency, and scaling (that is, the number of chips devoted to AI operations). For hardware, the key efficiency metrics for AI are related to the data center itself. Power Usage Effectiveness (PUE) measures how much power needs to be delivered to the data center to provide 1 watt to the chip. Over time, PUE is asymptotically approaching the ideal: 1 watt for 1 watt.
The other measure is “FLOPS per watts,” a measure of chip efficiency. It’s a ratio of how many floating-point operations per second (FLOPS), a measure of computation speed, can be achieved per 1 watt of input power delivered to the chip. According to the Green500 list of supercomputing efficiency, the record has improved to 72.7 billion FLOPS per watt, up from 4.4 billion FLOPS per watt in 2014.
And AI algorithms may be quickly leaving their most energy-hungry stage of development. The training phase, where core models are built, is power and space intensive while being location flexible, which is why training might happen out of country. After that comes the inference phase where core models are deployed, and that does not require as much power or space.
More on Artificial Intelligence: How AI Could Impact Manufacturing
So, the good news is that hardware efficiency and algorithm efficiency are moving in the right direction. Unfortunately, AI usage has been increasing even faster, which means the growth in AI demand is overwhelming all the efficiency gains.
On the other hand, energy accounts for less than 10 percent of the costs for AI systems. The real costs are for the chips themselves and the R&D staffs to develop and train the models. And though the energy requirements for AI are non-trivial, many of the early use cases, some of which are earning revenues or achieving results, are energy related.
More from the Magazine: Crunching Numbers, Sustainably
For instance, mining and exploration companies are using AI to comb the geological literature and core samples from around the world to accelerate the discovery or copper, cobalt, and other critical minerals. Fusion startups are deploying AI to accelerate their reactor designs, research labs are using it to speed up the discovery and testing of advanced battery materials, and utilities are relying on AI to extend the deployed life of critical assets like transformers. And the petroleum industry is finding uses for AI in optimizing oil and gas networks, gas lift, and enhanced refinery asset control.
The words—accelerating, optimizing, and life-extending—are typical phrases we use to capture the potential of AI. In these ways, the energy demands by AI will be more than offset by the energy savings. Fingers crossed.
Michael E. Webber is the Sid Richardson Chair in Public Affairs and the John J. McKetta Centennial Energy Chair in Engineering at the University of Texas at Austin. Webber said he did not write this essay with AI, but he probably should have done so because in that case it would have taken less time.