Video Games Offer Brain-Computer Interface Training Ground
Video Games Offer Brain-Computer Interface Training Ground
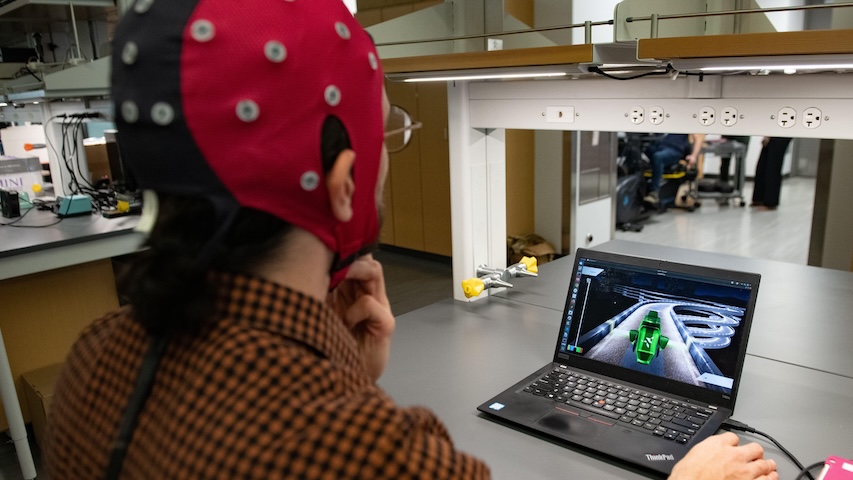
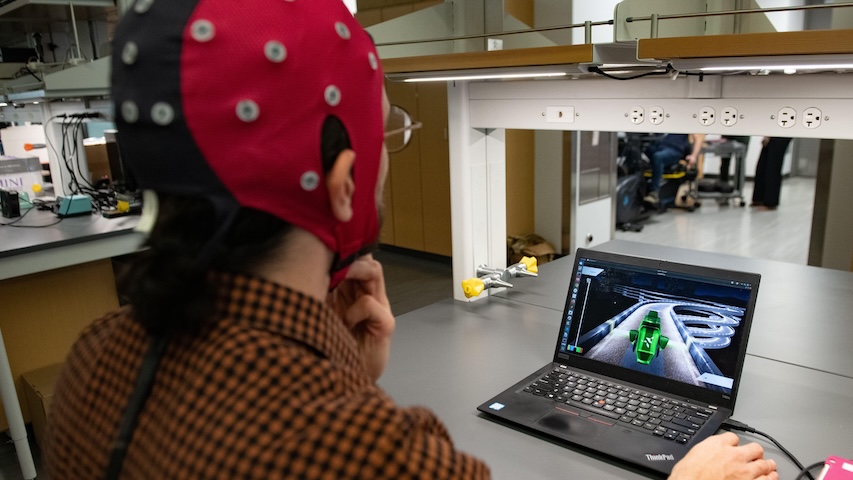
A calibration-free interface is not only making hands-free gaming a possibility but may lead to new ways to help individuals with motor disabilities.
Engineers at The University of Texas at Austin have developed a brain-computer interface (BCI) combined with machine learning that will allow just about anyone to don an electrode-equipped cap and get to playing games with their minds.
This research is part of an expansive effort to help improve the lives of people with motor disabilities.
What makes this interface significant is the fact that although most BCIs require extensive calibration for each individual user, the UT solution is calibration-free.
Training brains
About 20 years ago when he was working in robotics, José del R. Millán, a professor in the Cockrell School of Engineering’s Chandra Family Department of Electrical and Computer Engineering and Dell Medical School’s Department of Neurology, began to wonder how robots could interact with people who have severe motor disabilities and are unable to easily express intentions and commands. This spurred his interest in developing BCIs with a goal of someday building a brain-controlled robot.
Today, his team is not only developing a variety of BCIs, but new ways of training people to utilize these systems.
In a paper, “Transfer learning promotes acquisition of individual BCI skills,” published in PNAS Nexus earlier this year, Millán’s team outlined their development of the calibration-free BCI.
“So normally in the field of machine learning, because you need to recall those brain signals that you are recording, the assumption is that the sources that are generating your data are stationary. This is why you can get some data, build a model, and then deploy the model because your model will be analyzing the same kind of data that we used to build it," Millán said.
Become a Member: How to Join ASME
But brain signals are changing constantly, which means that any data gathered is endlessly evolving. This got Millán and his team of graduate students thinking about how they could design machine learning algorithms to support people learning how to use BCIs and help them learn how to control BCIs faster.
Just a few students are working on this particular project, which is complimented by several other ongoing projects in the lab, Millán said.
Some expert help
The BCI itself is relatively straightforward at first glance. Researchers fitted a cap to the brim with electrodes that gather a user’s electrical brain signals. Those signals are then sent to a computer, where a decoder translates those signals into actions within the video game.
But the problem is that people don’t all have the same “good” brain signals that will allow them to instantly have perfect control of a BCI, Millán said. “Learning to control a BCI is like learning anything—some people can learn quickly, while others learn slower,” he added.
This landmark study, as Millán calls it, doesn’t simply gather multitudes of data from multiple sources to teach the interface how to translate brain signals. Instead, the team trained one individual, or expert, who had good brain signals and was able to quickly and accurately learn how to use the BCI.
That expert’s data then served as the basis for the decoder, teaching the interface which signals meant which movements. That decoder then provided the basis for the system to translate signals from any user, making it possible for someone to use the BCI without a lengthy individualized calibration process.
“Then boom, everybody had good control from the very first day of training,” Millán said. “And furthermore, as we were hypothesizing, even though we were using the model of someone else, since we are working in a very high dimensional space during this learning, the modulations that people learn to generate were individual. Everybody was converging on something different.”
Mind Work: AI Helps Soldiers Bark Orders Telepathically to Robot Dogs
During the experiment, test subjects played two different games: one racing cars and another that required balancing two sides of a digital bar. The decoder enabled users to learn how to play both games simultaneously, even though each one required different levels of commands.
Why a video game? “Well, a game is funny,” Millán said. “This was more to motivate people because it’s funny.”
But there’s a practical reason as well, he added, that being a video game takes place inside a computer. Preparing experiments with upper limb exoskeletons, hand exoskeletons, or wheelchairs takes a lot of time and training.
The calibration-free BCI project featured 18 test subjects who had no motor impairments, but the team hopes to eventually test the system on individuals with motor impairments so that the BCI can be applied to larger groups in clinical settings.
This work is setting the foundation for future BCI innovations as well—a long-term goal is to develop BCI-controlled rehabilitation devices, or special mechanically designed robots that replicate all the articulations of the body, Millán said.
“We are also observing that long-term use of a brain-computer interface induces activity dependent brain plasticity,” which is the brain’s ability to grow and reorganize, Millán explained. So, these experiments are designed to both improve a patient’s brain function when learning how to use a BCI and improve a patient’s everyday life with BCI-controlled devices.
“On the one hand, we want to translate the BCI to the clinical realm to help people with disabilities,” he added. “On the other, we need to improve our technology to make it easier to use so that the impact for these people with disabilities is stronger.”
Louise Poirier is senior editor.