Nuclear Coolant Pump Monitoring Boosted with Wavelets
Nuclear Coolant Pump Monitoring Boosted with Wavelets
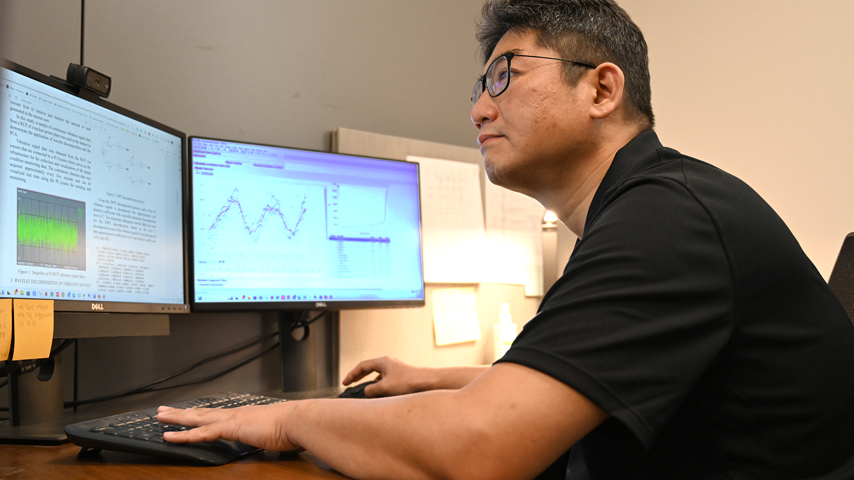
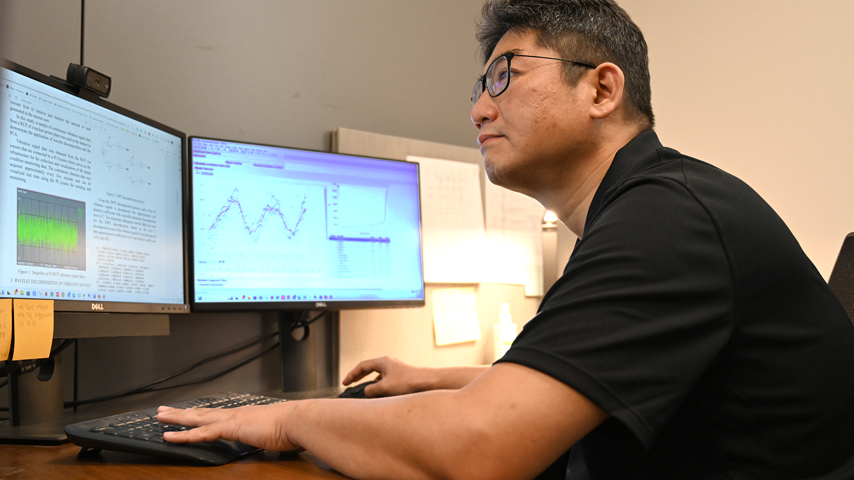
Researchers show discreet wavelet decomposition may offer better monitoring of nuclear coolant pumps.
Researchers at the University of Missouri are feeling confident their development of a new vibration signal analysis technique will lead to improved safety at nuclear power plants. The new technique relies on discrete wavelet decomposition of captured signals to monitor nuclear reactor coolant pumps. The wavelet analysis shows promise in capturing localized features and connecting them to environmental factors to help plant operators better understand what abnormalities in the patterns may mean.
Doctoral candidate and first author Benjamin Oguejiofor, along with Assistant Professor Kangwon Seo, both from the university’s Department of Industrial Systems Engineering, presented the initial results of their study at the 2023 Reliability and Maintainability Symposium earlier this year.
Both Seo and Oguejiofor focus on the role of predictive maintenance in operations. Nuclear power plants need constant monitoring and coolant pumps are especially important to a successful predictive maintenance plan. The collection of real-time vibration data via sensors and the analysis of that data is essential to identifying any deviations from desired parameters. This allows operators to make the proper adjustments for optimal reactor performance and power output. Of course, if a coolant pump malfunctions or fails, a much more serious situation could occur where heat from the nuclear reactor leads to a rapid increase in temperature and an eventual meltdown or other major safety event.
Become a Member: How to Join ASME
The idea to study the application of wavelet analysis of the vibration data arose during Oguejiofor’s day-to-day work at a local power plant. His work as an engineer there includes monitoring vibration data for any abnormal signals and ensuring an appropriate response is made to keep the plant running smoothly.
The power plant where Oguejiofor works currently uses vibration monitoring technology based on traditional fast Fourier transform (FFT). FFT converts the vibration signals into a spectrum that allows engineers to map them on a frequency spectrum. But vibration signals collected during a coolant pump monitoring can change rapidly and often contain localized peaks. That’s a feature not captured very well in FFT, according to Oguejiofor, making FFT analysis less valuable unless a fault pattern appears globally. Oguiejiofor and Seo thought there must be a better way.
“According to subject experts of nuclear power plant vibration monitoring, like Benjamin, vibration signals of a nuclear power plant often show more small bumps at some limited time range. We call it a local feature because it only appears at some part of the entire signal,” Seo explained.
Discrete wavelet transform (DWT) provides time-scale information, enabling extraction of features that vary with time. Though DWT itself is not new, the process of using it to monitor coolant pumps and connect the analysis of vibration signals to environmental factors “is an outcome of a combination of human knowledge and technology,” according to Seo.
Working with plant operators, the MU team obtained information and data on expected characteristics of an abnormal vibration signal. That information showed that in an abnormal status, an increased amplitude of vibration signal is expected and “wiggly small bumps” or peaks tend to appear at some local places.
More for You: Nuclear's Role in Combatting Climate Change Is Quantified
“Those timewave forms, they have a lot of peaks in their signal,” Oguejiofor said. “The wavelet captured those peaks very well. They can model those peaks very well.”
Of course, the signal is just that; a signal, a representation of a condition, Seo noted.
“What we are really aiming to do is… not only look at the signal itself but we are trying to identify the relationship of the signal with environmental factors,” Seo said.
Seo and Oguejiofor offered several examples of those factors, including temperature, motor current, or the flow rate.
The MU researchers decomposed the vibration signals into three different sub-bands with 12 features for each for a total of 36 features per vibration signal. Research results showed four principal components explain variability for about 96 percent of the total 36 features. With these four principal components explaining the variability researchers can focus on monitoring those remaining abnormal signals.
Editor's Pick: SMRs Are Promising but Disposal Issues Remain
“If we can successfully identify the relationship between this vibration signal and this environmental factor, then we may be able to find out the best optimal condition of this environmental factor, that it makes the vibration as the ideal shape we want to get,” Seo said.
Though they’re not yet actively involved in real world implementation, they’re excited for the potential of the wavelet analysis. Seo is working with other faculty at the university to explore how DWT may apply to other situations, such as measuring the different patterns or clusters of power usage.
“It’s a quite different application with vibration signal, but the same technique applies,” Seo said. Other future steps could include working with vendors to develop sensors that will be able to pull signals using the wavelet transform.
Seo said the team is continuing their work on collecting data on the environmental factors through the summer. The team aims to present these additional outcomes at the INFORMS annual meeting in Phoenix in mid-October. INFORMS bills itself as an international community of data science, analytics, and operations research professionals.
Nancy Kristof is an independent writer in Denver.
Doctoral candidate and first author Benjamin Oguejiofor, along with Assistant Professor Kangwon Seo, both from the university’s Department of Industrial Systems Engineering, presented the initial results of their study at the 2023 Reliability and Maintainability Symposium earlier this year.
Both Seo and Oguejiofor focus on the role of predictive maintenance in operations. Nuclear power plants need constant monitoring and coolant pumps are especially important to a successful predictive maintenance plan. The collection of real-time vibration data via sensors and the analysis of that data is essential to identifying any deviations from desired parameters. This allows operators to make the proper adjustments for optimal reactor performance and power output. Of course, if a coolant pump malfunctions or fails, a much more serious situation could occur where heat from the nuclear reactor leads to a rapid increase in temperature and an eventual meltdown or other major safety event.
Become a Member: How to Join ASME
The idea to study the application of wavelet analysis of the vibration data arose during Oguejiofor’s day-to-day work at a local power plant. His work as an engineer there includes monitoring vibration data for any abnormal signals and ensuring an appropriate response is made to keep the plant running smoothly.
The power plant where Oguejiofor works currently uses vibration monitoring technology based on traditional fast Fourier transform (FFT). FFT converts the vibration signals into a spectrum that allows engineers to map them on a frequency spectrum. But vibration signals collected during a coolant pump monitoring can change rapidly and often contain localized peaks. That’s a feature not captured very well in FFT, according to Oguejiofor, making FFT analysis less valuable unless a fault pattern appears globally. Oguiejiofor and Seo thought there must be a better way.
“According to subject experts of nuclear power plant vibration monitoring, like Benjamin, vibration signals of a nuclear power plant often show more small bumps at some limited time range. We call it a local feature because it only appears at some part of the entire signal,” Seo explained.
Discrete wavelet transform (DWT) provides time-scale information, enabling extraction of features that vary with time. Though DWT itself is not new, the process of using it to monitor coolant pumps and connect the analysis of vibration signals to environmental factors “is an outcome of a combination of human knowledge and technology,” according to Seo.
Working with plant operators, the MU team obtained information and data on expected characteristics of an abnormal vibration signal. That information showed that in an abnormal status, an increased amplitude of vibration signal is expected and “wiggly small bumps” or peaks tend to appear at some local places.
More for You: Nuclear's Role in Combatting Climate Change Is Quantified
“Those timewave forms, they have a lot of peaks in their signal,” Oguejiofor said. “The wavelet captured those peaks very well. They can model those peaks very well.”
Of course, the signal is just that; a signal, a representation of a condition, Seo noted.
“What we are really aiming to do is… not only look at the signal itself but we are trying to identify the relationship of the signal with environmental factors,” Seo said.
Seo and Oguejiofor offered several examples of those factors, including temperature, motor current, or the flow rate.
The MU researchers decomposed the vibration signals into three different sub-bands with 12 features for each for a total of 36 features per vibration signal. Research results showed four principal components explain variability for about 96 percent of the total 36 features. With these four principal components explaining the variability researchers can focus on monitoring those remaining abnormal signals.
Editor's Pick: SMRs Are Promising but Disposal Issues Remain
“If we can successfully identify the relationship between this vibration signal and this environmental factor, then we may be able to find out the best optimal condition of this environmental factor, that it makes the vibration as the ideal shape we want to get,” Seo said.
Though they’re not yet actively involved in real world implementation, they’re excited for the potential of the wavelet analysis. Seo is working with other faculty at the university to explore how DWT may apply to other situations, such as measuring the different patterns or clusters of power usage.
“It’s a quite different application with vibration signal, but the same technique applies,” Seo said. Other future steps could include working with vendors to develop sensors that will be able to pull signals using the wavelet transform.
Seo said the team is continuing their work on collecting data on the environmental factors through the summer. The team aims to present these additional outcomes at the INFORMS annual meeting in Phoenix in mid-October. INFORMS bills itself as an international community of data science, analytics, and operations research professionals.
Nancy Kristof is an independent writer in Denver.
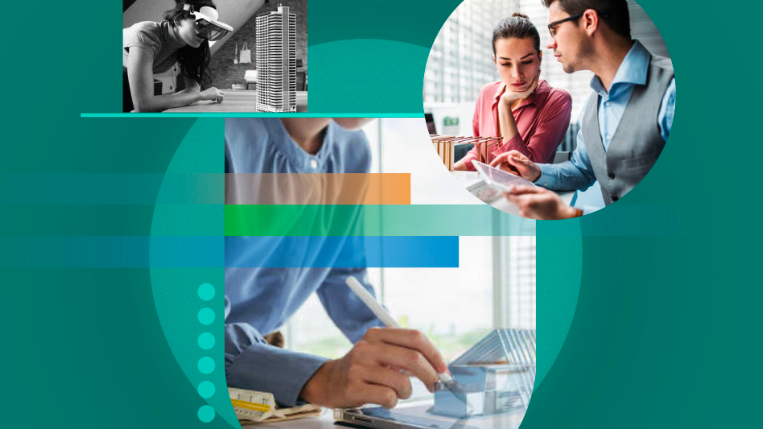
Pulse of the Profession: CAD in 2030
CAD technologies and their applications have been steadily evolving, ushering in a bevy of new approaches.