Machine Learning Applies to Pipeline Leaks
Machine Learning Applies to Pipeline Leaks
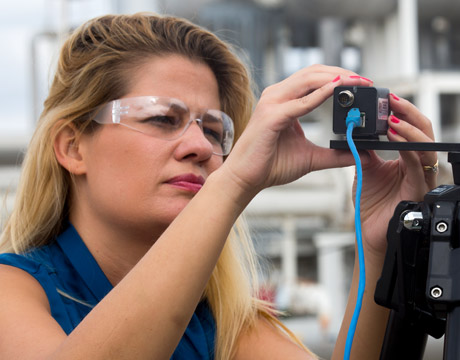
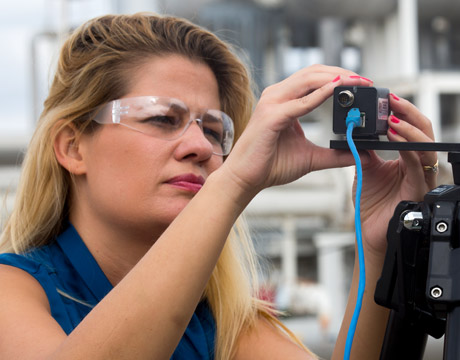
Maria Araujo leads the SWRI team developing an autonomous leak-detection system. Image: Southwest Research Institute
The Keystone pipeline that would move crude oil from Canada through the U.S. to a refinery in Texas has been controversial, but it would only be a fraction of the more than two million miles of pipelines moving oil and gas around the country. Many existing and proposed pipelines spark the same concerns from people as Keystone: the potential for leaks, especially those that go undetected for long periods of time.
Existing detection systems mostly spot large problems, often visually by inspectors walking or flying over a pipeline. Internal systems commonly used in the oil and gas industry rely on computational pipeline modeling, which searches for anomalies in flow and pressure. That works well for large leaks, but falls short in finding smaller ones, of up to one percent of pipeline flow, says Maria Araujo, a manager in the Intelligent Systems Division of the Southwest Research Institute.
Even such a small percentage adds up quickly. She notes that one percent of the flow of the Keystone pipeline is in the neighborhood of 8,000 gallons per day. To improve the efficiency of detection systems, Araujo leads a team taking the technology to the next level using sensors, artificial intelligence, and deep learning. She came to the problem of leak detection while working with machine learning for autonomously driven vehicles.
“We’re not adapting technology,” she says. “We’re using existing technology as building blocks. The problem is very different. With cars, you’re looking for objects. Here, you look for liquids. Gasoline and diesel are transparent to the human eye. How do you differentiate between substances?”
Actually, the system looks for a variety of liquids. To begin tackling the challenge, the SWRI team tested four optical sensors: thermal, optical, hyperspectral and short wave infrared. They eliminated hyperspectral and short wave infrared, keeping off-the-shelf thermal and optical systems.
There’s nothing unusual about using sensors for detecting leaks, but Araujo wanted to improve accuracy. So the SWRI team set out to adapt machine learning techniques, ultimately producing a multiplatform dubbed SLED, Smart Leak Detection System, that uses new algorithms to process images and identify, confirm or reject potential problems. Using feature extraction and classifier training methods, they taught computers to identify unique features across a wide range of environmental conditions.
“These algorithms thrive on lots of data,” says Araujo. The team produced and collected thousands of images of data such as gasoline, diesel fuel, mineral oil, crude oil and water on various surfaces, including grass, gravel, dirt and hard surfaces such as concrete. The images were shot from numerous angles and under varying conditions from full sunlight to clouds and darkness. “It’s hard to operate under different environmental conditions,” she adds. “We found if you train [the system] under certain conditions, it gets tripped up in others, especially shading. Being able to work under shading and different temperatures was a big challenge in modifying algorithms.”
The ability of the system to provide a reliable fingerprint of small leaks as well as identify non-leak situations greatly increases its accuracy. That’s important because one of the biggest problems in the industry is a false alarm, says Araujo. Pipelines wind their way across long and often remote or underground rights of way. Sending work crews to remote areas and shutting a pipeline down costs a significant amount of money, and operators can dismiss alarms if there were previous false alerts, she says.
SWRI further upgraded the system using deep-learning techniques. The team developed a deep convolutional neural network to process the tremendous amount of data to identify the hazardous liquids. Such techniques have been impractical in most cases, but advances and improvements in multi-core processing hardware are making it more common, say researchers. The final product is a fully autonomous system that can be used without human oversight, says Araujo.
It can be fitted to pumping station platforms along pipeline routes, often a high-risk location because of the number of valves and equipment that can break. The SWRI team also has installed and successfully tested the system on drones that can fly over long reaches of a pipeline.
“We simulated pipeline leaks with a high degree of replication to the real world,” she says. The work was done at SWRI’s Forth Worth, TX, campus, using existing piping and systems. The initial goal was to identify the difference between water and hazardous liquids, but it exceeded expectations by differentiating between gasoline, crude oil, mineral oil and diesel, as well as water.
Araujo now is working to adapt the technology to detect pipeline methane leaks in a program with the U.S. Department of Energy’s National Energy Technology Laboratory. The team is using infrared cameras to detect the spectral response of the gas. The deep-learning algorithm also must be reworked, a task she says is much more than an adaptation.
“This is a very significant ‘tweak’,” she says. “Now you are trying to detect a plume, something that shifts with the wind. It is a different problem.”
The goal is to produce an automated small-scale gaseous leak-detection system along the entire natural gas supply chain, including extraction, storage, distribution and transportation.
We’re using existing technology as building blocks. Gasoline and diesel are transparent to the human eye. How do you differentiate between substances?Maria Araujo, Southwest Research Institute