Giving Autonomous Vehicles 3D Vision
Giving Autonomous Vehicles 3D Vision
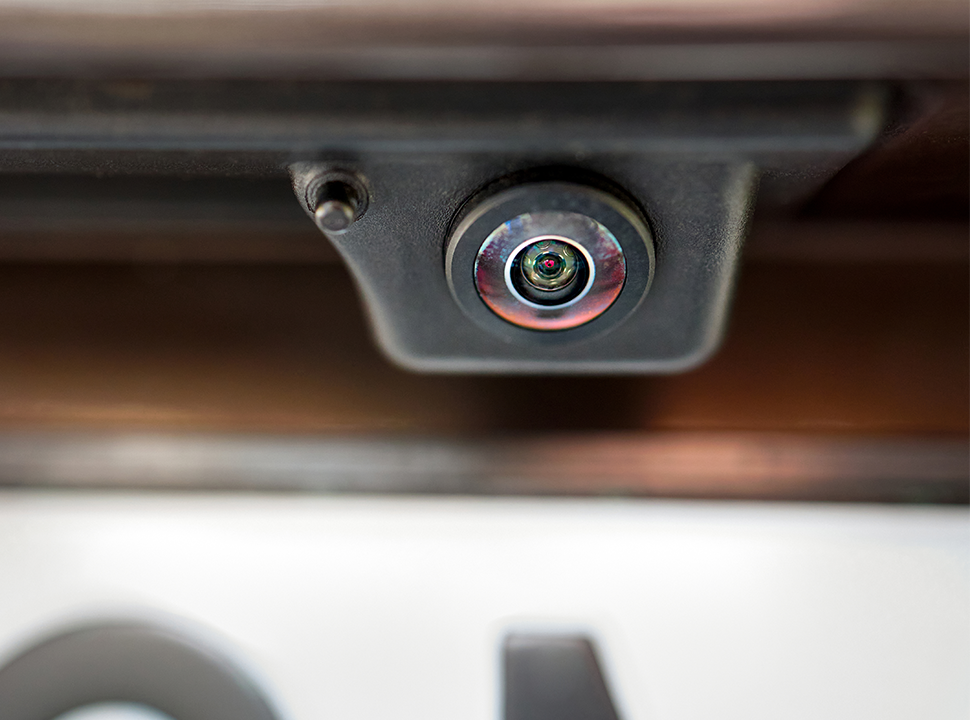
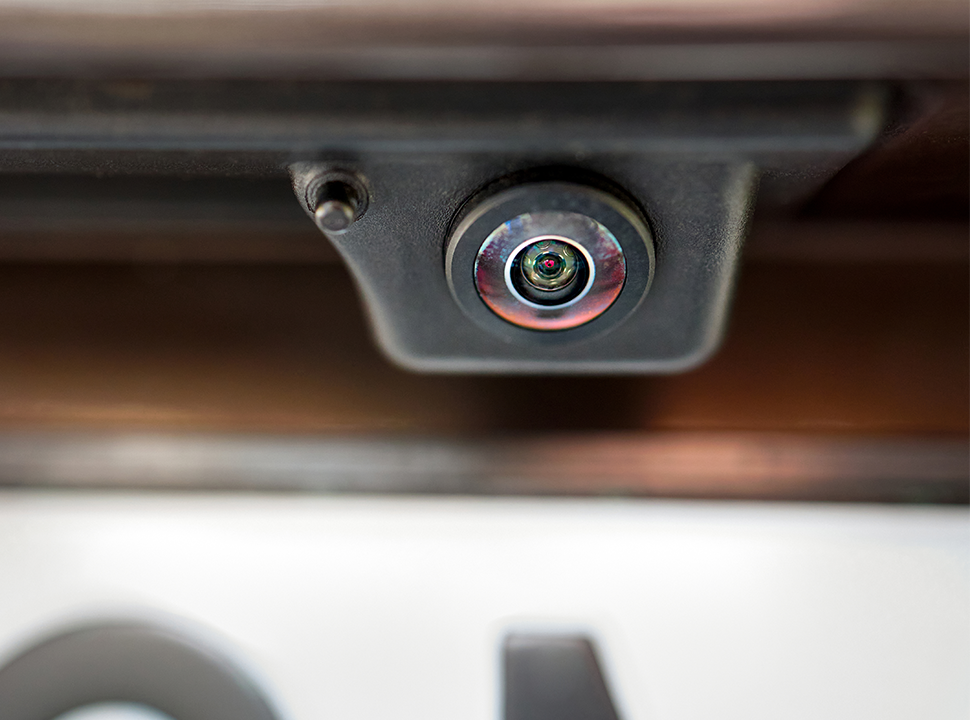
New statistical technique addes depth perception to camera images used by autonomous vehicles.
Moving through our three-dimensional world comes naturally to humans, but not so naturally for machines that navigate their worlds on their own volition.
Take, for instance, the autonomous vehicles and robots that use artificial intelligence to make second-by-second decisions about their environment. To do that, they depend on two-dimensional camera and video images and—to add that crucial third dimension—on Light Detection and Ranging (LIDAR), a laser measurement system that calculates the distance between objects. said Tianfu Wu, assistant professor of electrical and computer engineering at North Carolina State University.
LIDAR combines laser light pulses with other information captured by a vehicle to generates a three-dimensional view of vehicle surroundings, for instance the street on which the vehicle is traveling.
But LIDAR is expensive and could significantly drive up the cost of the self-driving vehicles that promise to eventually become an everyday part of municipal transportation services. What’s more, the expense means vehicles don’t offer a great deal of redundancy, as manufacturers limit the amount of sensors included on their vehicles.
Now, Wu and his colleagues have come up with a way to extract 3D data from 2D camera views. Future passengers may be thankful for the method, called MonoCon, which can cut a vehicle’s reliance on LIDAR and, in turn, slash operating costs while matching vehicle safety.
“AI programs receive visual input from cameras,” Wu said. “So if we want AI to interact with the world, we need to ensure that it is able to interpret what 2D images tell it about 3D space.”
Listen to the Podcast: Creating an Autonomous Driving Ecosystem
Wu’s team had been at work on AI for autonomous fixed-wing aircraft when they noted an important gap in the research. Most systems train AI by presenting it with 2D images and asking it to make decisions about its surroundings. The systems gradually learn how to improve its decision-making capabilities, but always within a 2D world.
With his background in statistics, Wu saw how the Cramér–Wold probability theorem—which proves joint convergence—could be applied to bring depth to flat camera images.
That theorem is at the heart of MonoCon, which identifies the 3D objects in 2D images, the stick in the middle of a flat road—and draws “bounding boxes” around them. The boxes look a little like kids’ efforts to draw boxes in 3D by connecting the corners of two overlaid squares.
More for You: Driverless Tractor for Hands-Free Farming
As with previous training methods, the AI system is given the 3D coordinates from each of a box’s eight corners and, from there is able to find the width, the length, and the height of each box. The system is then asked to estimate the distance between the center of the box, which offers what Wu calls “auxiliary information” needed to perceive depth.
In training, the system combines the three-dimensional measurements and the auxiliary information to estimate the dimensions of each bounding box and to predict the distance between the camera and the car.
Reader’s Choice: Automating the Risk Out of Farming
After each prediction, the digital trainers “correct” the AI by giving it the right answer after it’s made an incorrect estimate. Over time, the system gets better and better at identifying 3D objects, placing them in a bounding box, and estimating the dimensions of the objects, Wu said.
Autonomous vehicles will always need LIDAR, which is the most reliable method they have of moving quickly and accurately through three dimensions. But MonoCon is an economical way to cut the number of expensive sensors needed on each vehicle. It builds extra redundancy into the AI system, making it both safer and more robust, Wu said.
Jean Thilmany writes about engineering and science in Saint Paul, Minn.
Take, for instance, the autonomous vehicles and robots that use artificial intelligence to make second-by-second decisions about their environment. To do that, they depend on two-dimensional camera and video images and—to add that crucial third dimension—on Light Detection and Ranging (LIDAR), a laser measurement system that calculates the distance between objects. said Tianfu Wu, assistant professor of electrical and computer engineering at North Carolina State University.
LIDAR combines laser light pulses with other information captured by a vehicle to generates a three-dimensional view of vehicle surroundings, for instance the street on which the vehicle is traveling.
But LIDAR is expensive and could significantly drive up the cost of the self-driving vehicles that promise to eventually become an everyday part of municipal transportation services. What’s more, the expense means vehicles don’t offer a great deal of redundancy, as manufacturers limit the amount of sensors included on their vehicles.
Now, Wu and his colleagues have come up with a way to extract 3D data from 2D camera views. Future passengers may be thankful for the method, called MonoCon, which can cut a vehicle’s reliance on LIDAR and, in turn, slash operating costs while matching vehicle safety.
“AI programs receive visual input from cameras,” Wu said. “So if we want AI to interact with the world, we need to ensure that it is able to interpret what 2D images tell it about 3D space.”
Listen to the Podcast: Creating an Autonomous Driving Ecosystem
Wu’s team had been at work on AI for autonomous fixed-wing aircraft when they noted an important gap in the research. Most systems train AI by presenting it with 2D images and asking it to make decisions about its surroundings. The systems gradually learn how to improve its decision-making capabilities, but always within a 2D world.
With his background in statistics, Wu saw how the Cramér–Wold probability theorem—which proves joint convergence—could be applied to bring depth to flat camera images.
That theorem is at the heart of MonoCon, which identifies the 3D objects in 2D images, the stick in the middle of a flat road—and draws “bounding boxes” around them. The boxes look a little like kids’ efforts to draw boxes in 3D by connecting the corners of two overlaid squares.
More for You: Driverless Tractor for Hands-Free Farming
As with previous training methods, the AI system is given the 3D coordinates from each of a box’s eight corners and, from there is able to find the width, the length, and the height of each box. The system is then asked to estimate the distance between the center of the box, which offers what Wu calls “auxiliary information” needed to perceive depth.
In training, the system combines the three-dimensional measurements and the auxiliary information to estimate the dimensions of each bounding box and to predict the distance between the camera and the car.
Reader’s Choice: Automating the Risk Out of Farming
After each prediction, the digital trainers “correct” the AI by giving it the right answer after it’s made an incorrect estimate. Over time, the system gets better and better at identifying 3D objects, placing them in a bounding box, and estimating the dimensions of the objects, Wu said.
Autonomous vehicles will always need LIDAR, which is the most reliable method they have of moving quickly and accurately through three dimensions. But MonoCon is an economical way to cut the number of expensive sensors needed on each vehicle. It builds extra redundancy into the AI system, making it both safer and more robust, Wu said.
Jean Thilmany writes about engineering and science in Saint Paul, Minn.