Computer Chips Could Reduce AI's Energy Footprint
Computer Chips Could Reduce AI's Energy Footprint
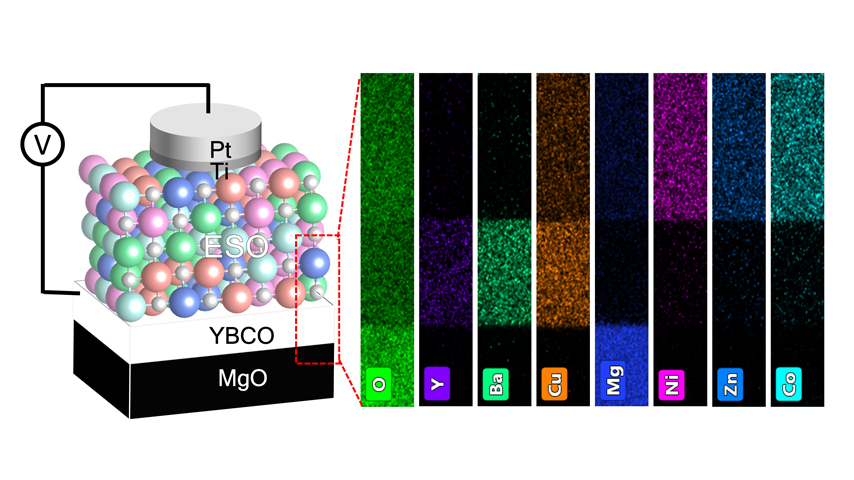
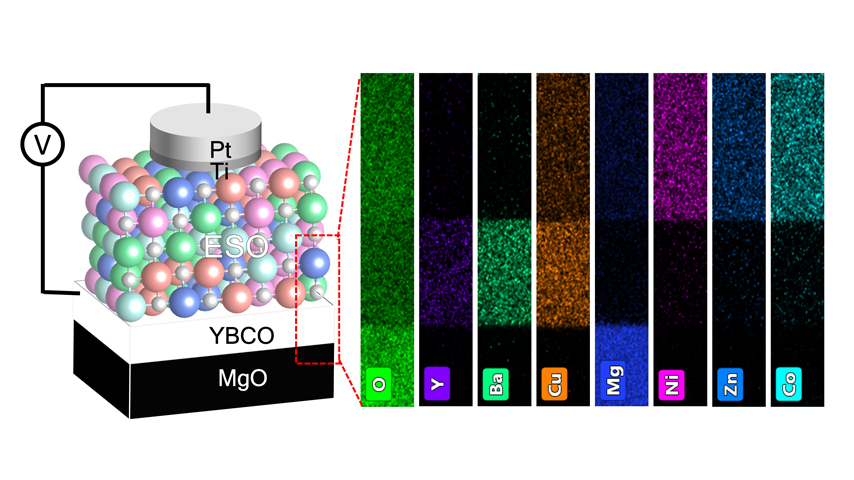
An answer to skyrocketing energy use driven by AI may come from new chip materials.
The presence of artificial intelligence (AI) in our daily lives seems increasingly inescapable-─it’s becoming a standard in internet searches and online reviews, serving as bots, or pushing AI-generated imagery and videos—and its growth shows no sign of slowing down. As its impact on our everyday lives unfolds, AI is also affecting rising energy demands.
A ChatGPT query process uses 10 times as much electricity as a Google search, according to International Energy Agency data cited by Goldman Sachs, which also says AI is expected to drive a projected 160 percent increase in energy demand by 2030.
And while a recent Forbes article pointed to increased power consumption per computing chip as contributing to the increased demand, two new recently published studies show the promise of using a new type of material to improve chip energy efficiency six-fold. The materials are called entropy-stabilized oxides, or ESOs, and their use in memristors, which are used to control the flow of electrical current in a circuit, saves energy by eliminating the need for external memory storage.
The team leading the work includes Oregon State University researcher Chae Sieun and Sangmin Yoo, a postdoctoral researcher at IMEC USA and the University of Michigan. Sieun participated as a doctoral student at Michigan before joining the faculty at OSU.
More for You: Understanding the Limits of Machine Learning
“The basis is this colocation of memory and processor,” Sieun said.
The first study, published in May in Nature Electronics, was followed by an August update published in Applied Physics Letters.
Sieun has focused her career on researching materials while Yoo offers device expertise. They also happen to be married. Conversations over the dinner table turned to ways to use ESOs to improve energy efficiencies of computer chip processing.
“I was looking for applications where these materials are very useful, and then my husband introduced this device where he can tune device properties based on the type of information,” she said.
ESOs are emerging materials in ceramics and are made up of a solid solution of more than five different cations, differentiated by high-entropy oxides (HEOs). Memristors typically have been constructed using thin films of metal oxides, such as titanium dioxide (TiO2), but the multiple elements in ESOs provide more flexibility.
“The advantage of this material is that I can tailor material properties very easily and very finely,” said Sieun.
Discover the Benefits of ASME Membership
Conventional computing systems use an architecture where memory and processing units are segregated, creating “severe latency and energy consumption issues,” when the data must move between the two segregated components via a data bus, according to the introduction on the team’s latest study. This creates a “memory bottleneck” as the amount and frequency of data moving back and forth increases.
“[With] the traditional computer, we have memory and processors and they are two different components,” Seiun said. These new memristors operate in ways similar to those found in biological neural networks.
“A memristor has this combined function that people say is like a mimicking of the brain,” she added. Like the brain, memristors don’t use an external memory source, which means energy is not lost moving data back and forth.
“It takes time to move the data between memory and processor, here, we are saving this energy, the power and time that it takes to transfer data,” Sieun said.
Single-crystalline ESO memristors show the ability to delicately tune internal transport dynamics through composition control of point defects. This allows implementation of neural network systems for task-specific machine learning applications that consume less energy. ESOs allow for better control of memristor device properties, such as enhanced reliability and predictability. Information storage in the form of easily programmable electrical conductance makes ESOs suitable for data processing without a need to transfer data between the processor and memory.
“This is the perfect application,” she said. “We can process data and store data at the same time.”
Energy Blog: Is AI Too Power-Hungry for Our Own Good?
The combination of materials that use less energy for AI processing, along with an increasing reliance and interest in powering data centers with renewable energy sources is promising according to the studies team, though work remains to be done.
“I think this material is so brand new that for the industry or adoption, it might take a long time,” said Sieun, adding that there are many private companies working on advancing this type of technology. Future work on tuning a broader range of internal dynamics and higher functionality will make ESO memristors even more promising for sustainable AI computing, according to the study’s authors.
The team’s work was supported by funding from the National Science Foundation and led by researchers at the University of Michigan. The collaboration also included researchers from the University of Oklahoma, Cornell University, and Pennsylvania State University.
Nancy Kristof is a technology writer in Denver.